PhD position – Modelling and analysis of carbon-climate interactions in northern forest ecosystems
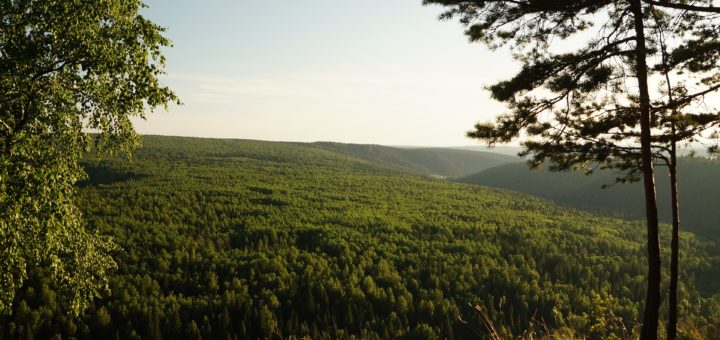
Title: Modelling and analysis of carbon-climate interactions in northern forest ecosystems
Institute: University of Edinburgh and University of Helsinky
Position Term: 4 years
Application Deadline: 31 October 2020
Summary
This project focuses on the carbon-climate interactions of boreal forests, combining multiple data sources with process models to understand and predict the evolution of these ecosystems. The project is joint between the Universities of Edinburgh and Helsinki.
Project background
Climate change is occurring most rapidly at high latitudes, which are regions with large C stocks in soils and extensive forest cover. But the response of boreal forests to climate change remains poorly understood. Some of the key uncertainties are related to below-ground allocation and respiratory losses of carbon. While ample sources of different types of environmental and ecosystem data are becoming available, the data need to be integrated and interpreted with models to provide complete ecosystem analyses, and to underpin predictions. This project will test alternate representations of processes of forest C balance in ecosystems models from Finland and the UK. Testing will use rich sets of observations from field sites in Finland and the UK, supplemented by targeted new field measurements. The research will determine the role of process uncertainty in determining future C sources and sinks in high latitude forests. Modelling will be used to explore the interactive role of climate change, management (e.g. harvest frequency) and disturbance on C storage. The student will be based in Edinburgh and spend summers in Finland undertaking field measurements, interacting closely with research groups in both countries.
Research questions
The overarching objective of the project is to advance our understanding of climate-C coupling in forest ecosystems and the representation of this in a range of process-based ecosystem models. To this end, we will address the following questions (i) how do alternate representations for internal processing of C in forest ecosystem models compare?; (ii) how does process uncertainty affects forecasts of C sink/source strength?; (iii) what is the climate and management sensitivity of different C processes and their interactions?
Methodology
The project builds on existing modelling resources, field observation data, and model-data fusion expertise, at Edinburgh and Helsinki. The project will use two forest C models, one developed in Edinburgh (DALEC, Williams et al. 2005) and the other in Helsinki (PREBASSO, Valentine and Mäkelä 2005, Minunno et al. 2019). These models differ in their representation of key C cycle processes – photosynthesis, allocation, turnover, phenology, respiration, decomposition, mineralisation. They also differ in the climate sensitivity of these processes. Further, there are >15 different versions of DALEC, varying in the number of C pools, and process representation. This diversity of model structures, complexity, and climate sensitivity can be explored, quantified and tested across boreal forest systems. The project will determine the strengths and weaknesses of the process representation and their coupling, providing insights to advancing theory and improve model structure.
Models and observations can be combined, so that the observations calibrate and validate the models, while the models provide a test of theorised links between observed processes. Bayesian approaches are revolutionising the science of model-data fusion (Williams et al, 2005; Van Oijen 2017). Bayesian approaches recognise that models should be calibrated probabilistically, based on the uncertainty in observational data. Bayesian analysis also allows for model comparisons and using the most probable combinations of models for making future predictions. Bayesian approaches ascertain that uncertainty is attached to parameter estimates, and the interactions between processes are clarified. These properties make Bayesian analysis an efficient tool for model development and testing.
Training
A comprehensive training programme will be provided comprising both specialist scientific training and generic transferable and professional skills. The PhD student will be able to join large and thriving PhD student communities in both institutions. For instance, Edinburgh hosts a large Doctoral Training Programme in Ecology and Environmental Sciences (https://www.ed.ac.uk/e4-dtp) and a Centre for Doctoral Training in Satellite Data for Environmental Science (https://eo-cdt.org). The student can connect to both Programme and Centre, taking advantage of their training courses and networking opportunities. The student will also join Prof Williams’ Global Change Ecology Lab, working with its students and post-doctoral researchers to develop skills and collaborate on research into global forest C cycling. In Helsinki the student will join the Ecosystem Process group at the Department of Forest Sciences.
Requirements
An undergraduate degree in natural sciences to at least upper second level (or equivalent), with strong quantitative skills including programming in languages such as R or python. It would be advantageous to have a MSc degree. The applicant should have a strong motivation to apply numerical skills to understanding ecosystem processes.
Additional information at University of Edinburgh website
Info Autori
Istituto per i Sistemi Agricoli e Forestali del Mediterraneo (ISAFOM)
Consiglio Nazionale delle Ricerche (CNR)